Winter into Spring Doctoral School "Understanding, Controlling, and Designing human-AI interactions"
March 24-26, 2025, in Freiburg/Breisgau, Germany
As AI systems increasingly influence high-risk contexts in areas such as healthcare, HR, law enforcement, and critical infrastructure, many discussions about the ethical and human-centered design of those systems center around the topics of understandability and controllability. This workshop offers a platform for networking, collaboration building, and topical exchange for early-career researchers (PhD, PostDocs, Junior Research group leaders) working on interdisciplinary research at the intersection of work and organizational psychology/human factors/management and computer science, likely augmented with philosophical, ethical, and legal perspectives.
Why Attend?
• Get feedback on your research: The workshop offers an opportunity to engage with other ECRs and with the keynote speakers from diverse backgrounds. You will have the chance to exchange ideas and receive feedback on your work.
• Find collaborators: There will be time dedicated to work on research ideas and collaboration with your peers to tackle open research questions.
• Embrace the complexity: This workshop aims to highlight the complexity of perspectives in research on human-AI interaction and tries to let participants reflect on the interdisciplinary perspectives of their research.
Example Key Topics:
• Explainability, Transparency, and Understandability of AI
• Controllability, Responsibility, and Accountability in relation to AI
• Human Oversight of AI-based systems
• Work Design and AI
• Organizational Behavior and AI
• Tradeoffs in the design of AI-based systems
• Ethical implications of using AI
• The intersection between emerging legislation and human-AI research
Is this workshop something for me?
This workshop is ideal for PhD students and early-career researchers who are:
• Working on interdisciplinary research at the intersection of work and organizational psychology/human factors/management and computer science
• Interested in the topics of XAI, human control, human oversight, work design and related topics.
• Eager to network with peers and experts in the field, share research ideas, and engage in collaborative problem-solving around the critical issues of AI transparency and human oversight.
Workshop Details
Keynote speakers doctoral school (see details below):
- Elena Glassman, Harvard University
- Zana Buçinca, Harvard University
- Johann Laux, University of Oxford
Pitch sessions: In these sessions, you will present your work in short talks. You will receive feedback from your peers, the organizers and the keynote speakers.
• Joint project workout sessions: In these sessions, you will have the opportunity to develop cooperation ideas with your peers. On the last day of the workshop, there will be the opportunity to present these ideas and receive feedback.
Participation
Participation is free of charge - you will only need funding for the travel and accommodation in Freiburg.
If you are interested in participating, please submit a max. 500 words Abstract on the research / topic / idea / position that you want to present during a short pitch talk in the workshop until the 16th of December 2024 via the following online form here:
We will select the submitted ideas based on fit to the workshop ideas. If there are more submissions than are free slots during the workshop, we will select on a first come first served basis.
Link to pictures on external page linkedin:

Elena Glassman, Harvard University
Title:
Leveraging Theories of Human Cognition to Build Reliable Tools from Unreliable AI
Abstract:
AI is powerful, but it can make choices that result in objective errors, contextually inappropriate outputs, and disliked options. This is especially critical when AI-powered systems are used for context- and preference-dominated open-ended AI-assisted tasks—like ideating, summarizing, searching, sensemaking, and the reading and writing of text or code. We need AI-resilient interfaces that help users notice and recover from AI choices that are not right, or not right for them given their goals and context. We have derived design implications from key theories of human cognition to help us build more AI-resilient interfaces and reliable tools from unreliable AI. This talk will walk through two new systems that demonstrate this approach: CorpusStudio, an AI-powered writing environment, and MOCHA, a tool for co-adaptive machine teaching.
Bio:
Elena L. Glassman is an Assistant Professor of Computer Science at the Harvard John A. Paulson School of Engineering & Applied Sciences, specializing in human-computer interaction. Prior to that, she was a postdoctoral scholar at UC Berkeley, and obtained a BS, MEng, and PhD in Electrical Engineering and Computer Science from MIT. She has been named a Stanley A. Marks & William H. Marks Professor at the Radcliffe Institute for Advanced Study and a National Academy of Sciences Kavli Fellow. Her work has been funded by the NSF, private industry, the Berkeley Institute for Data Science, and the Sloan Research Fellowship. This work has received Best Paper and Honorable Mention awards at top-tier human-computer interaction research venues.
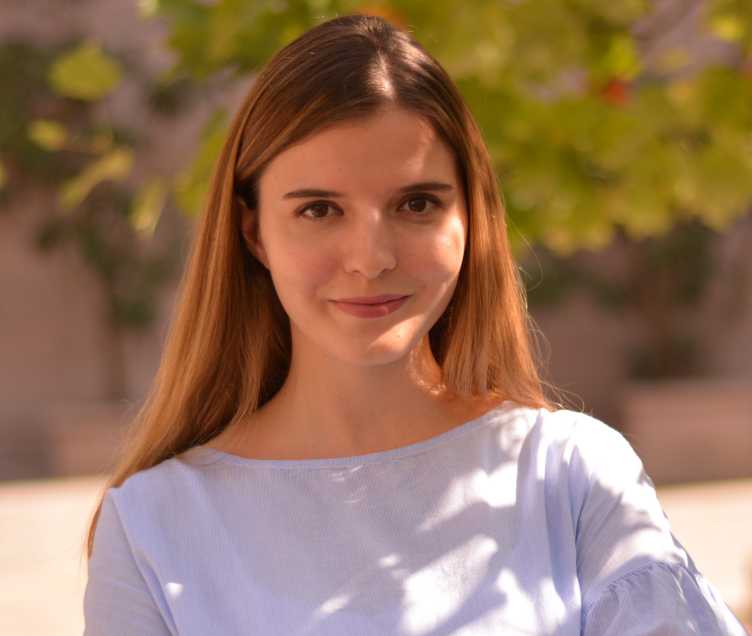
Zana Buçinca, Harvard University
Title:
Value-Aligned Human-AI Interaction
Abstract:
The anticipated large-scale deployment of AI systems in knowledge work will impact not only productivity and work quality but also workers' values and workplace dynamics. I argue that how we design and deploy AI-infused technologies will shape people's skills and competence, their sense of agency, collaboration with others, and even the meaning they derive from their work. I design human-AI interaction techniques that complement people and amplify their values in AI-assisted work. My research focuses on (1) understanding how people make AI-assisted decisions and (2) designing novel interaction paradigms, explanations and systems that optimize human-centric outcomes (e.g., human skills) and output-centric outcomes (e.g., decision accuracy) in AI-assisted tasks. In this talk, I will present a suite of interaction techniques I have introduced to optimize AI-assisted decision-making. These include cognitive forcing interventions that reduce overreliance on AI, adaptive AI support that enables human-AI complementarity in decision accuracy, and contrastive explanations that improve both decision accuracy and users’ task-related skills.
Bio:
Zana Buçinca is a PhD candidate in Computer Science at Harvard working at the intersection of human-AI interaction and responsible AI. Her research integrates cognitive and social science theories to design novel human-AI interaction techniques that complement workers and amplify their values in AI-assisted tasks. Her work has been recognized with the IBM PhD Fellowship, a Siebel Scholarship, and a Best Paper Award at IUI 2020. Zana has been named a Rising Star in AI by the University of Michigan, a Rising Star in Management Science & Engineering by Stanford, and one of the Top 10 Most Inspiring Women in STEM by UNDP Kosovo.
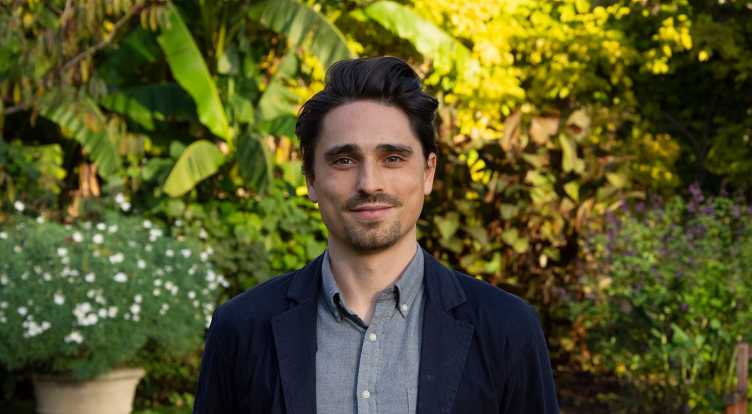
Johann Laux, University of Oxford
Title:
Behavioural Assumptions in AI Regulation
Abstract:
The legal regulation of Artificial Intelligence ("AI") makes an astonishing number of explicit and implicit assumptions about how humans will interact with AI systems and how AI systems will "behave" in the wild. This talk questions the empirical basis of these behavioural assumptions. It argues that many are empirically unfounded, conceptually misguided and result in an anthropomorphisation of AI systems. Regulators should instead aim to better integrate empirical findings from disciplines such as psychology, human-AI interaction research, and sociology in their attempts to govern AI.
Bio:
Johann Laux is a lawyer and social scientist working on AI governance. He is the Principal Investigator of the Emerging Laws of Oversight research project at the Oxford Internet Institute, University of Oxford. His work combines law & policy analysis with social science methods and investigates issues such as the effectiveness conditions of human oversight of AI, the trustworthiness of AI systems, and the market dynamics of algorithmic personalization.